Understanding Deep Learning and Its Applications
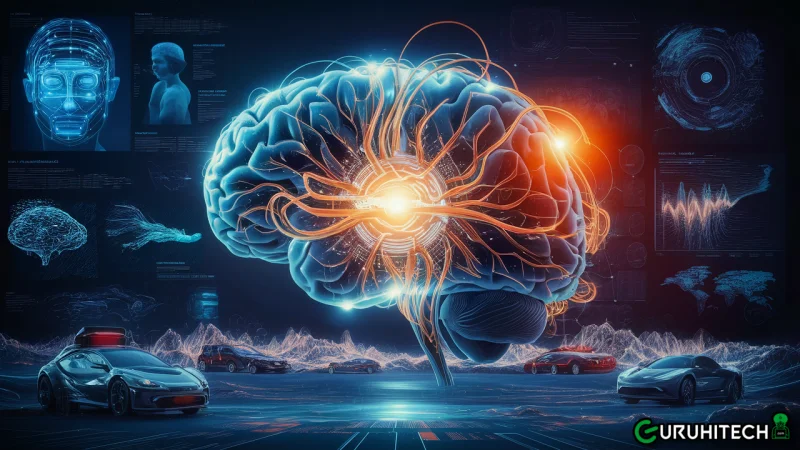
Deep learning, a subset of machine learning, has made significant strides in recent years, revolutionising various industries with its ability to process and analyse vast amounts of data. By mimicking the human brain’s neural networks, deep learning algorithms can identify patterns and make decisions with unprecedented accuracy. This article explores the fundamentals of deep learning and its diverse applications across different sectors.
What is Deep Learning?
Deep learning involves training artificial neural networks to recognise patterns in data. These neural networks consist of layers of interconnected nodes, or neurons, that process information and learn from it. Each layer extracts specific features from the input data, allowing the network to build a hierarchical representation of the information. This approach enables deep learning models to handle complex tasks such as image recognition, natural language processing, and speech synthesis.
In order to enhance your knowledge and skills there are many online courses and free certification courses, ensuring you remain at the forefront of this exciting and dynamic field. Embrace the power of deep learning and explore its potential to drive innovation and improve outcomes in your industry.
Key Components of Deep Learning
- Neural Networks: The backbone of deep learning, neural networks are composed of multiple layers, including an input layer, hidden layers, and an output layer. Each neuron in a layer is connected to neurons in the previous and next layers, allowing information to flow through the network.
- Activation Functions: These functions determine whether a neuron should be activated or not, based on the input it receives. Common activation functions include the sigmoid, tanh, and ReLU (Rectified Linear Unit).
- Training Algorithms: Deep learning models are trained using large datasets and optimisation algorithms such as gradient descent. The training process involves adjusting the weights of the connections between neurons to minimise the error between the predicted and actual outputs.
- Loss Functions: These functions measure the difference between the predicted and actual outputs, guiding the training process. Common loss functions include mean squared error and cross-entropy loss.
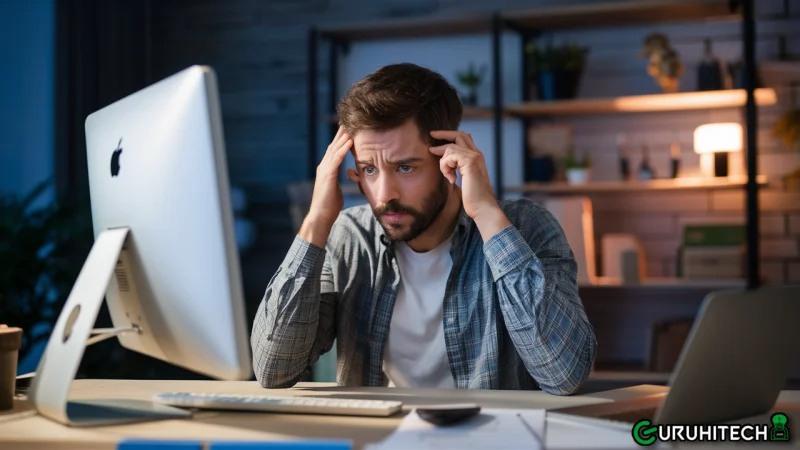
Applications of Deep Learning
- Image Recognition: Deep learning has revolutionised image recognition, enabling applications such as facial recognition, object detection, and medical imaging. Convolutional neural networks (CNNs) are particularly effective in processing visual data, allowing computers to identify patterns and features in images with high accuracy.
- Natural Language Processing (NLP): Deep learning has significantly advanced NLP, powering applications like chatbots, language translation, and sentiment analysis. Recurrent neural networks (RNNs) and transformers are commonly used to process and generate human language, enabling machines to understand and interact with text and speech.
- Autonomous Vehicles: Deep learning is a critical component of self-driving cars, helping them perceive and navigate their environments. By processing data from sensors such as cameras, lidar, and radar, deep learning models enable vehicles to detect objects, recognise traffic signs, and make real-time driving decisions.
- Healthcare: In the healthcare sector, deep learning is used for tasks such as diagnosing diseases, predicting patient outcomes, and personalising treatment plans. For instance, deep learning models can analyse medical images to detect tumors or predict the progression of chronic diseases.
- Financial Services: Deep learning is transforming financial services by enhancing fraud detection, automating trading strategies, and improving customer service. By analysing vast amounts of financial data, deep learning models can identify fraudulent activities, predict market trends, and offer personalised financial advice.
- Entertainment: Deep learning is used in the entertainment industry to create realistic animations, generate music, and recommend content. For example, streaming services use deep learning algorithms to analyse user preferences and suggest movies or shows that match their interests.
Staying Updated with Deep Learning: Importance of Education
Given the rapid advancements in deep learning, staying updated with the latest trends and techniques is crucial for professionals in the field. One effective way to enhance your knowledge and skills is through online courses. These courses provide a structured learning path and cover a wide range of topics, from the basics of neural networks to advanced deep learning architectures.
For those looking to gain formal recognition of their skills, free certification courses are an excellent option. These courses often include hands-on projects and assessments, allowing you to apply what you’ve learned in practical scenarios. Completing a certification course not only boosts your resume but also demonstrates your commitment to continuous learning and professional development.
Conclusion
Deep learning is transforming numerous industries with its ability to analyse and interpret complex data. From image recognition and natural language processing to autonomous vehicles and healthcare, the applications of deep learning are vast and varied. As the field continues to evolve, staying updated with the latest advancements is essential for professionals.
Ti potrebbe interessare:
Segui guruhitech su:
- Google News: bit.ly/gurugooglenews
- Telegram: t.me/guruhitech
- Facebook: facebook.com/guruhitechweb
- Twitter: twitter.com/guruhitech1
- Instagram: instagram.com/guru_hi_tech/
- Threads: threads.net/@guru_hi_tech
- GETTR: gettr.com/user/guruhitech
- Rumble: rumble.com/user/guruhitech
- VKontakte: vk.com/guruhitech
- MeWe: mewe.com/i/guruhitech
- Skype:Â live:.cid.d4cf3836b772da8a
- WhatsApp: bit.ly/whatsappguruhitech
Esprimi il tuo parere!
Ti è stato utile questo articolo? Lascia un commento nell’apposita sezione che trovi più in basso e se ti va, iscriviti alla newsletter.
Per qualsiasi domanda, informazione o assistenza nel mondo della tecnologia, puoi inviare una email all’indirizzo guruhitech@yahoo.com.